How can you measure a person's beliefs? What about their risk attitudes? What methods could we use to minimise reporting bias?
In our Measures Matter series, we unpack measurement tools used to study psychology and behaviour in research evaluations. The goal of the series is to share knowledge from our research and learnings from our experiences in the field with researchers interested in behavioural economics, psychology and psychiatry. We provide step-by-step instructions on how to replicate or build on our measurement approaches.
Non-cognitive skills (NCS) are a group of behavioural and attitudinal traits or abilities that cover a range of abilities such as conscientiousness, perseverance, and self-control (see the Glossary for more examples). Using appropriate tools to measure NCS is crucial to learning accurately about the levels of these skills in different populations. This post outlines the process of validating likert-type scales used to measure NCS. To ensure scales perform well, this validation process should be performed during piloting and as part of quality checks conducted during data collection
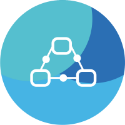
In face to face surveys, there may be concerns about social desirability bias resulting in under-reporting of what respondents perceive as negative behaviours and over-reporting of positive behaviours. We piloted some measures for the objective measurement of education investment assets in the endline survey of a Randomised Control Trial in Kenya (Orkin et al., 2020). This post provides details on each measure and the issues encountered in the setting.
The Centre for the Study of African Economies runs a popular Coder's Corner series providing advice on managing data and code used in analysis. Below are links to a few of their posts which might be particularly useful for researchers in behavioural economics, psychology and psychiatry.
The interests of researchers and policymakers often extend beyond a simple average treatment effect when evaluating interventions in randomised experiments. Exploring heterogeneous treatment effects, or average treatment effects by subgroups and covariates, can provide useful answers to a variety of important questions. This post explores using machine learning methods for inference on heterogeneous treatment effects.
When we analyse the results of an experiment, we are often interested in understanding what the treatment effects are on sub-groups. This type of sub-group analysis is usually estimated using in-sample information on the relationship between the outcome of interest and the covariates in the control group to predict outcome for all groups without treatment. However, this procedure generates substantial bias due to overfitting. This post discusses a method for overmining this bias.
Including control variables in regressions can substantially increase the statistical power of your analysis. However, deciding which control variables to select is arbitrary. Pre-analysis plans allow researchers to credibly commit to a set of controls, yet these controls might turn out to be suboptimal ex-post. Regularisation techniques deal with this problem and ensure that you make the most of your existing data.
Extensive robustness checks have become a requirement for empirical research. This often leads to Online Appendices with hundreds of result tables that are very hard to digest for readers and referees. Stata16’s speccurve command written by Martin Eckhoff Andresen is an easy to use command that facilitates the generation of specification curves. A specification curve plots a large number of regression coefficients and confidence intervals sorted by estimated impact from different specifications that allow the assessment of robustness in a single figure.
How can mediation analysis be useful in an experiment that has a behavioural component? With multiple follow-ups on behavioural characteristics and socioeconomic variables, researchers can use mediation to test whether socioeconomic outcomes in later rounds can plausibly be explained by changes in the psychological variables at intermediate follow-up rounds after the behavioural intervention.
Measuring psychological outcomes can be difficult when the constructs we are interested in are unobservable (e.g., the Big Five personality traits) or very costly and time consuming to measure (e.g., clinical depression). Factor analysis is a statistical technique used widely by psychologists and social scientists. It enables us to test if a given set of measures captures an underlying, unobservable construct (factor). This helps us to select and verify our measurement instruments.
Field experiments in (behavioural) development economics have become increasingly complex. Many trials test whether cost-effective behavioural additions to more traditional interventions and rigorous analysis of heterogeneous treatment effects across sub-groups has become the norm. This post shows how you can create publication style balance and summary tables taking into account these complexities.
The following glossary offers common terminology and definitions of behavioural concepts cited in Mind & Behaviour research work.
This glossary evolves with our study themes. If you have any feedback or would like to propose additional measures we can review, please get in touch: mbrg@bsg.ox.ac.uk